
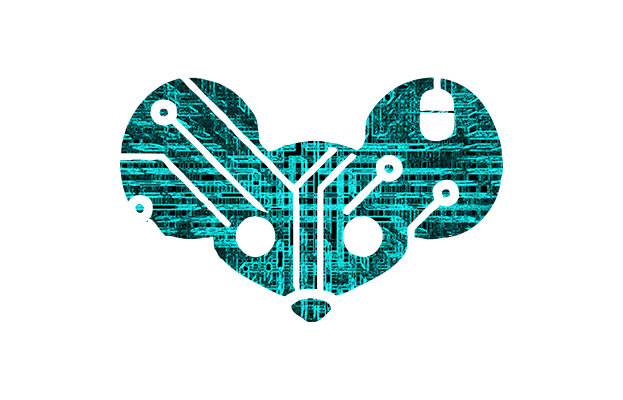
Postdoc in engineering research - we’re using machine learning to predict chemical properties relevant to combustion, speeding up the discovery of cleaner liquid fuels as we transition away from fossil fuels!
Postdoc in engineering research - we’re using machine learning to predict chemical properties relevant to combustion, speeding up the discovery of cleaner liquid fuels as we transition away from fossil fuels!
I use a few used Dell Optiplex 7050 Micros, they’re great for the price (and have a small footprint too!)
Edit: for storage I have a HP MicroServer Gen. 10 plus
Self-hosting lemmy.blue!
YouTube TV and Spotify. There’s a workaround for everything else!
No problem, happy to help out the fediverse!
Very cool, I’ll do some digging myself!
Interesting - it would be even more interesting if they provided some metrics as to how much time is being saved compared to RSS.
What’s worse:
Meanwhile Kim Dotcom, the founder of Megaupload, is continuing to fight the U.S. charges and threat of extradition. He has said he expects his former colleagues to testify against him as part of the deal they struck.
Ortmann was sentenced to 2 years and 7 months while van der Kolk was sentenced to 2 years and 6 months. Each had faced a maximum sentence of 10 years in prison but argued they should be allowed to serve their sentences in home detention.
Does the punishment fit the “crime”?
He’s gonna run Twitter into the ground like you would your favorite car
+1 for running TrueNAS in a VM, I’ve got one running in Proxmox. Make sure to enable hardware passthrough so TrueNAS has direct access to your drives!
My workflow for setting up a Lemmy instance goes something like this:
+1 for Docker, specifically Docker Compose. Lemmy probably isn’t the right container to learn Docker with, but once you have the fundamentals down spinning up Lemmy was pretty seamless.
TL;DR, I throw a bunch of molecules at a pile of linear algebra, and hope predicted values line up with known experimental values; then I use the pile of linear algebra on novel molecules.
There’s a bit more to it than that, like how to represent molecules in a computer-readable format, generating additional input variables (molecular characteristics), input variable down-selection and/or dimensionality reduction, the specific ML models we use (feed-forward MLPs and graph convolution nets), and how to interpret results as they relate back to combustion.
From a broad perspective, our work is just a small part of a larger push from the Department of Energy to find economically-viable alternative liquid fuels. ML speeds up the process of screening candidate molecules, for example those found in bio-oil resulting from pyrolizing and catalytically-upgrading lignocellulosic biomass or other renewable sources. Our colleagues don’t have to synthesize large samples of many molecules just to test their properties and determine how they will behave in existing engines (a very costly and time-consuming process), instead we predict the properties and behaviors to highlight viable candidates so our colleagues can focus on analyzing those.
These papers (1, 2, 3) best outline the procedures and motivations for this work. PM me if you can’t get access and I’ll send you them!